Sales teams dedicate a lot of their time to forecasting. Yet, over 50% of sales leaders question the accuracy of their sales forecasting efforts. Getting your estimates right is vital to your future business decisions.
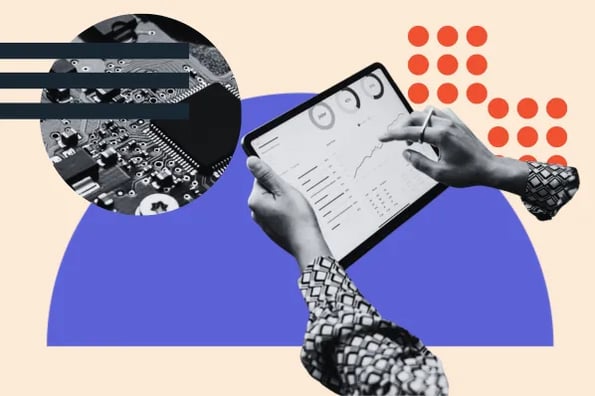
Since sales forecasting is a rather complex and time-consuming process, organizations seek ways to improve it, with many turning to machine learning.
In today’s article, we’re going to focus on machine learning sales forecasting. We will tell you what it is, its benefits, and the main sales forecasting techniques.
Table of Contents
- What is machine learning?
- Machine Learning and Sales Forecasting
- 6 Machine Learning Sales Forecasting Techniques to Know
What is machine learning?
Machine learning (ML) is a subset of data science and artificial intelligence (AI). ML trains algorithms to enhance accuracy and “learn” the same way human scientists would throughout a data project. Machines are taught to recognize their mistakes in previously produced work fully autonomously, without any human verification.
To create a well-functioning machine learning model, data scientists ‘feed’ it with a sample of data from a larger, high-quality database. Next, they train the model and check its output until they’ve made sure that it generates accurate information. After the verification stage, they can use ML for data circumstances without clear outcomes.
Machine learning is much more cost-effective and efficient than processes requiring ongoing human moderation. As a result, leaders throughout industries are adopting this model.
Machine Learning and Sales Forecasting
Sales forecasting is a difficult process. As many as 80% of sales organizations admit to having a forecast accuracy not greater than 75%. Is there anything that businesses can do to improve it? They can – turn to machine learning. It uses neural networks, a machine learning algorithm that identifies various patterns in historical data.
Machine learning generates accurate sales predictions based on time-series analysis, regression analysis, and decision trees. Since machine learning can analyze huge data sets, its predictions are much more accurate than those performed by humans.
The Benefits of Using Machine Learning in Sales Forecasting
Here are some advantages you can anticipate if you introduce ML into your sales forecasting process.
Better Sales Forecasting Accuracy
Machine learning models can process data and generate results with a speed impossible to achieve through manual, human work. This means whenever your sales team needs to plan for the months or years ahead, they can rely on their ML-powered analysis.
If you train an ML model on your real-life sales data (ideally, a large volume of information from various points in time), then you can rely on its prognoses’ accuracy.
Providing New Insights into Customer Behavior
The quest for improving the sales pipeline and closing more deals is never-ending. As machine learning can go over large amounts of data quickly, its role in boosting sales revenue is invaluable. How so? It reveals new patterns and insights into customer behavior.
Thanks to these, sales teams can spot new leads, build targeted sales campaigns, and predict which services or products will be in high demand.
Saving Time and Resources
Machine learning eliminates the need for manual data entry, manipulation, and analysis, significantly speeding up sales forecasting. These tasks are not only time-consuming but also prone to errors. Instead of dedicating their time to these mundane tasks, sales teams can focus on more strategic work that brings more value to the business.
What’s more, AI has excellent reporting capabilities. It creates clear and concise reports that can be nicely displayed on a dashboard, along with some metrics. This allows sales professionals to better understand and communicate the forecasts and make more accurate business decisions. They can modify their plans and focus on the most profitable opportunities or activities.
Spotting New Insights Through Uncovering Patterns
Traditional sales forecasting methods used by humans rely on comparing two variables in what is known as linear regression. IBM provides a great example — you could spot a relationship between your sales representatives’ sales and their years of experience.
While this is incredibly useful, machine learning models can get into far more advanced analyses. Often, this can reveal trends previously hidden in hindsight.
If you use an ML model, you could use your awareness of the high sales results–seniority factor and further find out that reps with the best results make twice as many outbound calls as others. Or, that they make most of their calls between 10 a.m. and 12 p.m., before their contact people go for lunch.
These are just a few hypothetical situations, but they prove just how sophisticated your forecasting techniques can get with the right ML model.
6 Sales Forecasting Techniques to Know (and Where Machine Learning Applies)
Let’s now look at six sales forecasting techniques that sales teams can use in their work.
Gut Feel Forecasting
As suggested by its name, gut-feel forecasting relies on your salespeople’s intuition. You ask them how they feel about their current deals, which ones they think they will manage to close, and when. As you can imagine, the accuracy of this method is rather low.
Smaller organizations often use this approach. Such businesses entirely rely on their sales pro’s experience and gut feeling, instead of seeking answers based on data.
Almanac Method
The Almanac method uses historical data to predict future performance. While this approach is more effective than the previous one as it’s based on facts and data, it’s not ideal. It doesn’t account for any market or macroeconomic changes that happen.
For example, using data from 2019 to predict what 2020 would look like would be a big mistake. 2020 was tumultuous because of the COVID-19 pandemic, which caused massive changes in customer behavior. As 2020 was nothing like 2019, basing sales predictions on historical data would be useless.
The Almanac method might work for companies that have access to a lot of historical data. The more data they have, the higher the chance of getting an accurate forecast.
Funnel Forecasting
Funnel forecasting is about taking a closer look at your sales funnel performance. What’s your win rate? How long is your average sales cycle? What’s the total volume of your sales pipeline? Getting answers to these questions will help you predict your future sales.
Let’s use an example to better understand how this method works. Let’s say your sales cycle is three months, and your average win rate is 30%. If you have 12 opportunities in the pipeline worth $350,000 each, you can safely assume that your sales forecast for the quarter will be $1,260,000.
The accuracy of funnel forecasting will depend on the coverage of your pipeline, as well as its health and how up-to-date it is. You can use this method if your sales process is long. With simple, short sales cycles, it won’t work as effectively.
Portfolio Forecasting
Portfolio forecasting is a mix of several sales forecasting strategies. You take into account both quantifiable insights like historical data and less ‘tangible’ aspects like years of experience, opinion, and potential market risks.
The goal for this sales forecasting method is to account for all possible factors – i.e., the entire ‘portfolio.’
For instance, a sales leader could turn to a model for the upcoming quarter that:
- Looks at the number of existing sales-qualified leads (SQLs) and calculates the probability of converting marketing-qualified leads (MQLs) to SQLs.
- Refers to historic sales results (for the last quarter and/or the same quarter the previous year).
- Takes their sales reps’ ‘gut feelings’ into account (for example, how likely they are to upsell to the key accounts they manage).
- Comparing a specific situation to similar scenarios in the past.
- Looking at market trends, including the financial situation of their target customers.
If the methods that worked in the past aren’t as promising for the current forecast, the sales leader can brainstorm ways that could help minimize a drop in revenue.
Multivariate Regression Analysis
As mentioned earlier, so-called ‘linear’ regression methods rely on comparing two objects or data points directly related to one another.
Meanwhile, multivariate regression sales forecasting is a statistics method where you look at multiple dependent variables and analyze them against one another. Their relationships can become linear after you establish how variables link to each other.
To understand how it works, let’s refer to an example by MyGreatLearning. It can be hard to predict the weather in June by simply comparing it to historical data for that month. There are other factors, i.e., variables, like a rise or drop in overall pollution, rainfall, or drought — all of which might be harder to predict due to global warming.
If we put this into a sales analogy, the different variables that will affect your sales could include not only historical data but also:
- Supply chain disruption.
- Manufacturing capacity (for example, the introduction of robotics).
- Inflation rate and change in pricing.
- Brand reputation (for instance, any successful marketing campaigns or online brand crises).
You’ll likely agree that this seems like an immensely powerful model. Unfortunately, there’s a “catch.” For multivariate regression sales forecasting to be accurate, you need to ensure that your data is of high quality. The more informational gaps or chaos in your datasets, your results are less reliable.
Machine Learning and AI
Machine Learning sales forecasting is the absolute best method for large-scale analyses. It’s the most versatile and powerful solution of all, as it can refine itself over time.
You can model your ML to reflect any (or all) of the traditional models and use your experience to tell which factors should be given the highest relevance. Your sales representatives can all contribute to your ML engines by powering them with their quantitative and qualitative information.
Once trained and verified in the initial testing stage, your ML can function as an independent assistant. Whenever it spots any new behavioral patterns or predicts potential risk factors, you and your team will be the first to know and, ultimately, adjust.
Machine Learning Can Take Your Sales Forecasting to a New Level
Machine Learning is bringing unprecedented speed and accuracy into sales forecasts. By incorporating it into your sales strategy planning, you and your team can react dynamically to any market changes — some of which your competitors might not even be aware of.
Look at HubSpot’s sales forecasting software if you’d like to learn more about how to increase your forecast accuracy and transform sales operations.